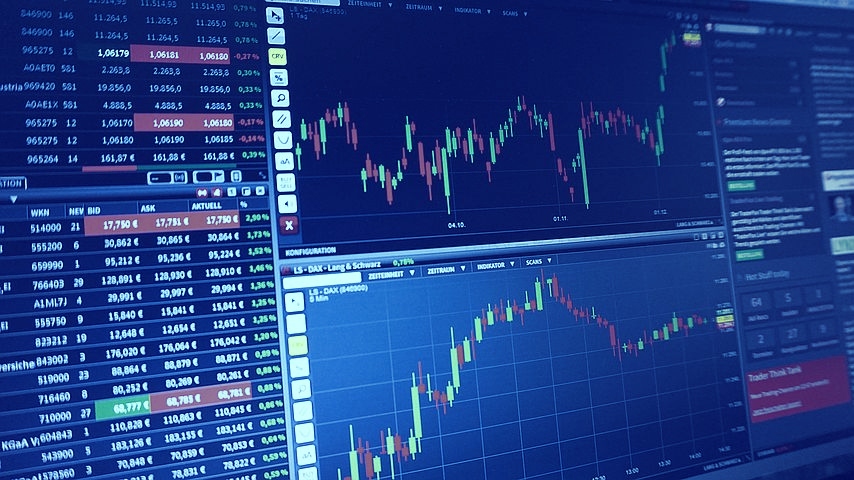
Feature Selection
As part of the research used, for example, in the StockPicker application we have been researching the selection of variables enterings. When there are too many variables, the model has a worse ability to generalize and therefore is less robust and more prone to errors.
- If you need to extract information from your data, do not hesitate to contact us.
Why (goal)
- Better understanding the data.
- Better understanding a model.
- Reducing the number of input features
Feature importance can provide insight into the dataset and show which features may be the most relevant to the target. This interpretation is important for domain experts and could be used as the basis or benchmark for gathering more and different additional data.
Feature importance can provide insight into the model. The most important features are calculated by a predictive model that has been fit on the dataset. Inspecting the importance score provides insight into that specific model and which features are the most important for it.
Feature importance can be used to improve a predictive model. This can be achieved by using the importance scores to select those features with the highest scores. This is a type of feature selection and can simplify the problem that is being modeled, speed up the modeling process (deleting features is called dimensionality reduction.
What (key points)
- linear machine learning algorithms fit a model where the prediction is the weighted sum of the input values
- all of these algorithms (linear regression, logistic regression and regularized extensions such as Lasso, Ridge and Elastic net) find a set of coefficients to use in the weighted sum in order to make a prediction
- these coefficients can be used directly as a crude type of feature importance
How (procedure)
- fit regression model on the regression dataset and retrieve the coeff_ property that contains found for each input variable
- the coefficients can provide the basis for a crude feature importance score
- this assumes that the input variables have the same scale or have been scaled prior to fitting a model
Bar chart of Elastic Net Regression as Feature Importance Scores